Study finds generative AI’s large language models (LLMs) may not directly replicate human preferences but offer valuable insights. The authors studied whether LLMs can accurately mimic human responses on consumer preference surveys.
Key Takeaways:
- Directly using LLMs in place of human respondents for preference elicitation may be misleading.
- LLMs can generate hypotheses about factors influencing consumer decisions for testing with humans.
BALTIMORE, MD, July 29, 2024 – Generative artificial intelligence (AI) platforms such as Open AI’s GPT-3.5 and GPT-4 have provided many new ways for researchers to better understand consumer behaviors and preferences, but one question that has yet to be answered is whether these large language models (LLMs) can accurately mimic human responses on matters of consumer preference.
Two researchers from the University of Washington have found that although current iterations of LLMs may not directly replicate human preferences, they can offer valuable insights into factors that influence consumer decision-making across different contexts and languages.
The research study is published in the INFORMS journal Marketing Science. The peer-reviewed article is called “Can Large Language Models Capture Human Preferences?” and is authored by Ali Goli and Amandeep Singh, both of the University of Washington.
“Our research shows that directly using LLMs to elicit preferences in place of human respondents could be misleading,” says Goli. “However, we found that LLMs can play an important role in generating hypotheses that can help researchers understand the underpinnings of heterogeneity in preferences across contexts and customer segments.”
The authors examined how LLMs process intertemporal choices – decisions between immediate and delayed rewards – across multiple languages. They found that although LLMs didn’t accurately replicate human patience levels, they did capture interesting variations across languages with different future tense structures.
“By analyzing the text generated by LLMs in different contexts, we were able to identify factors that might influence decision-making, such as risk perception, opportunity cost and urgency,” says Singh. “This approach can help researchers formulate hypotheses about what drives consumer preferences, which can then be tested with human subjects.”
The researchers employed a novel “chain-of-thought” approach, prompting the LLMs to explain their reasoning. This method, combined with topic modeling, revealed how different factors were weighted in various decision contexts.
“Our analysis showed that as the time delay between options increased, discussions about risk and uncertainty became more prevalent, while considerations of opportunity cost decreased,” explained Singh. “Similarly, when higher interest rates were offered, LLMs focused less on opportunity costs and more on immediate needs.”
These patterns parallel how human decision-makers might allocate mental resources across various factors, including urgency, uncertainty and opportunity cost. For instance, the results can help explain why humans have different discount rates over different time horizons.
“While LLMs may not directly replicate human preferences, our findings indicate they can be valuable tools for generating hypotheses about the factors underlying consumer behavior,” Goli added. “This approach could lead to more targeted and insightful human subject research in the future.”
The study’s findings suggest a pathway for using LLMs to enhance our understanding of consumer preference patterns, potentially leading to more nuanced research approaches in areas such as product development, marketing strategies and consumer education, particularly for products involving intertemporal trade-offs like mortgages, investments and insurance services.
About INFORMS and Marketing Science
Marketing Science is a premier peer-reviewed scholarly marketing journal focused on research using quantitative approaches to study all aspects of the interface between consumers and firms. It is published by INFORMS, the leading international association for operations research and analytics professionals. More information is available at www.informs.org or @informs.

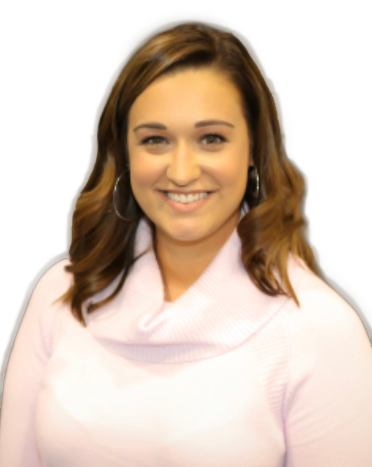
Media Contact
Ashley Smith
Public Affairs Coordinator
INFORMS
Catonsville, MD
[email protected]
443-757-3578